Did you know that over 70% of businesses struggle to leverage AI effectively? This staggering statistic points to a significant issue in the modern corporate landscape: as industries evolve and the digital transformation accelerates, the inability to successfully integrate AI technologies can hinder growth and innovation. The complexity of AI, combined with existing organizational obstacles, poses challenges that many businesses are unprepared to face.
This article aims to highlight the challenges businesses encounter with AI integration and showcase how addressing these issues can foster transformative solutions. We will delve into the common pain points associated with AI adoption, gain insights from industry leaders about their experiences, and illustrate how successful AI implementation can reshape operational models across sectors.
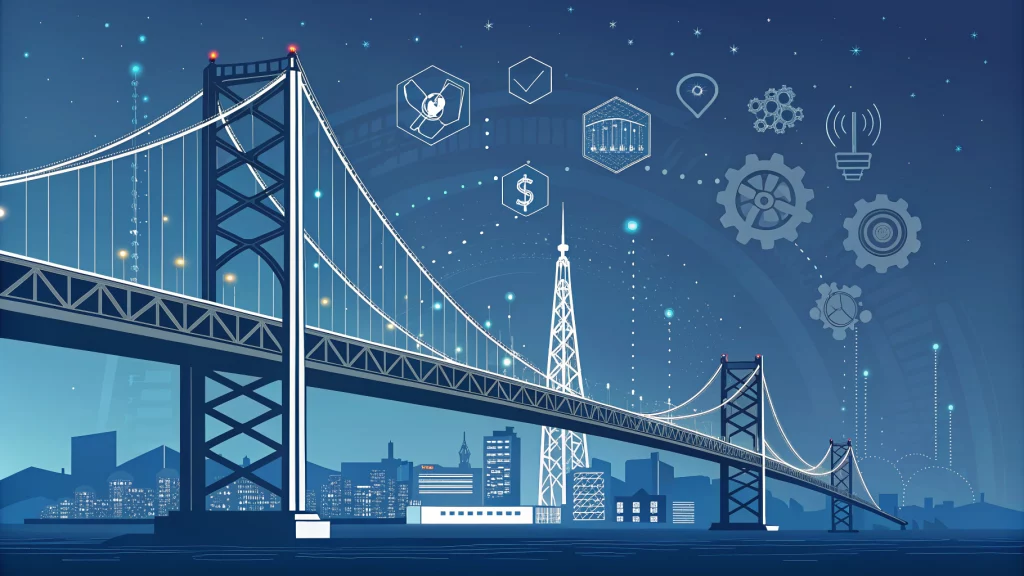
Identifying Common Challenges in AI Integration
Lack of Understanding and Knowledge Among Staff
One of the primary hurdles organizations face in their AI integration efforts is a profound lack of understanding and knowledge among staff. Many employees, particularly those not working directly with technology, lack a foundational understanding of AI capabilities and limitations. This gap in knowledge can lead to unrealistic expectations, resistance to new technologies, and ultimately, failure to effectively utilize AI systems. Businesses need to invest in training programs that empower their workforce to engage confidently with AI tools. Companies like Google have long invested in continuous learning initiatives, providing their employees with workshops and online courses to foster a culture of tech-savvy professionals.
Resistance to Change Within Organizational Culture
Another significant challenge is the cultural resistance to change within organizations. Employees often perceive AI as a threat rather than an opportunity, fearing job displacement or disruptions in their traditional workflows. This mindset can lead to pushback against AI initiatives, limiting the potential for innovation. To combat this resistance, leaders must focus on change management strategies that emphasize the value of AI as a collaborative tool. IBM, for example, has successfully implemented its AI solutions by championing an internal campaign that emphasizes collaboration between humans and machines, thereby alleviating fears of job loss.
Data Quality and Availability Issues
AI thrives on data; however, many organizations struggle with insufficient data quality and availability. Poor data can lead to inaccurate AI predictions and further complicate the integration process. Companies often operate on legacy systems that don’t communicate effectively or lack the data infrastructure necessary for AI to glean meaningful insights. Businesses should conduct thorough data audits and invest in modernizing their data architecture. Capital One is an exemplary case, having rebuilt its data strategy to focus on quality and accessibility, ultimately enabling more effective AI-driven decision-making.
Integration with Existing Systems and Processes
Lastly, many companies face challenges when attempting to integrate AI with their existing systems and processes. Legacy systems can be incompatible with modern AI solutions, leading to inefficient workflows and poor user experiences. A strategic approach to integration, including a phased rollout and thorough testing, is essential for mitigating these issues. For instance, during its AI implementation, Siemens set up a pilot program to test AI capabilities in a controlled environment before a full-scale rollout, ensuring compatibility and functionality before widespread adoption.
Insights from Industry Leaders
Key Strategies for Successful AI Integration
Drawing insights from industry leaders reveals effective strategies for successful AI integration. One of the most crucial strategies is fostering a culture of data-driven decision-making. Enabling employees at all levels to understand and utilize data helps build a more robust AI implementation. Leaders also emphasize the importance of collaboration between cross-functional teams, ensuring that technical staff and business units work together throughout the AI integration process. According to Sundar Pichai, CEO of Google, a common success factor for AI projects is establishing clear collaboration between engineers and business owners to create solutions that genuinely address user needs.
Real-World Examples of Successful AI Implementations
Many industry leaders offer real-world examples that demonstrate successful AI integration. For instance, Netflix uses AI algorithms to analyze viewer preferences, driving personalized recommendations that keep subscribers engaged. This clever use of data not only enhances user experience but also supports Netflix’s content strategy. In another instance, Unilever implemented AI for predictive analytics in supply chain management, resulting in a significant reduction in inventory costs and improved operational efficiency.
Common Pitfalls to Avoid Based on Leadership Experiences
Leaders often caution against several pitfalls related to AI integration. A common mistake is underestimating the time required for training both staff and AI systems. For example, in the case of General Electric (GE), initial AI implementation phases felt rushed, leading to inadequate system training and early failures. Another pitfall is failing to establish clear, measurable goals for AI projects. Without defined objectives, it becomes challenging to measure success and return on investment (ROI). Companies such as Deloitte have developed frameworks to help organizations set realistic AI objectives that align with broader business goals, encouraging successful long-term outcomes.
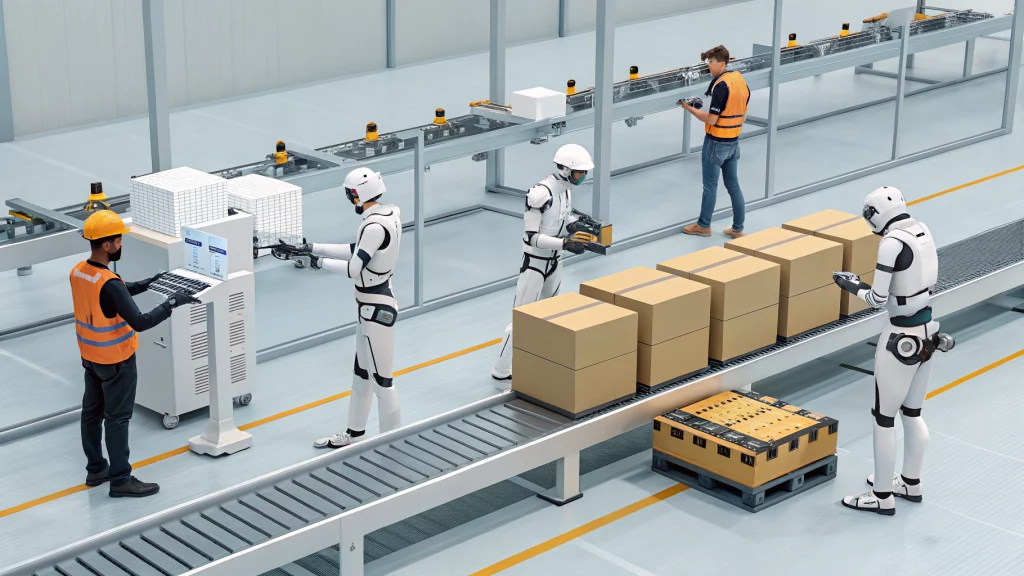
Transformative Solutions Provided by AI
Enhancing Operational Efficiency
AI technologies can substantially enhance operational efficiency by automating repetitive tasks and optimizing workflows. For example, robotic process automation (RPA) is increasingly adopted to streamline tasks such as invoice processing and data entry, freeing human resources for higher-value activities. Adobe, for instance, leveraged RPA to automate a significant portion of its administrative functions, resulting in reduced turnaround times and improved employee satisfaction.
Improving Decision-Making Capabilities
AI also transforms decision-making capabilities by providing predictive insights based on vast datasets. This level of analysis can help leaders make more informed strategic decisions quickly. For instance, Amazon harnesses the power of AI through its recommendation engine, which not only personalizes user experiences but also influences inventory and marketing strategies based on real-time customer behavior analysis. This ability to forecast trends and consumer preferences positions Amazon favorably in a highly competitive market.
Driving Innovation in Product Development
AI-driven innovations are propelling significant advancements in product development cycles. Machine learning algorithms can analyze customer feedback and performance data to suggest iterative improvements or entirely new product lines. For example, Tesla utilizes AI to enhance its self-driving technologies by processing data from its fleet of vehicles, allowing for rapid innovation in its offerings. By leveraging AI, companies can innovate faster and respond more adeptly to consumer demands.
Case Studies Showcasing Successful AI Outcomes
Numerous case studies showcase the transformative outcomes of AI implementations. For instance, Ford leveraged AI for predictive maintenance in its manufacturing processes, increasing uptime and reducing production costs. Similarly, Coca-Cola harnessed AI for customer engagement, leading to improved marketing strategies and customer loyalty. These case studies highlight the potential benefits of effective AI adoption, from improving operational efficiency to driving innovative solutions in product and service offerings.
Navigating the Future of AI in Business
Predictions from Experts Regarding AI’s Evolving Role
As industries continue to evolve, AI’s role is predicted to expand even further. Experts forecast a world where AI will become embedded into nearly every aspect of business operations, from customer service chatbots to advanced data analytics. For example, ABI Research anticipates that AI-driven robots in manufacturing will increase significantly, leading to more efficient production processes. Business leaders must be prepared for this accelerated integration, ensuring that their teams are equipped with the necessary skills to leverage these technologies.
The Importance of Continuous Learning and Adaptation
In a rapidly changing technological landscape, the importance of continuous learning cannot be overstated. Organizations must prioritize ongoing education and skill development for their workforce to keep pace with AI advancements. Companies like Accenture are already implementing continuous learning programs that focus on upskilling employees to handle emerging technologies, creating a proactive workforce that can adapt to new challenges effectively.
Future Trends Impacting AI Adoption Across Industries
Several trends are anticipated to impact AI adoption across various sectors. The rise of low-code and no-code platforms will likely democratize AI, allowing non-technical staff to harness AI for their business needs. Additionally, there’s a growing emphasis on ethical AI, as businesses seek to ensure that AI applications are developed and implemented responsibly. As AI continues to permeate industries, organizations must navigate these trends to remain competitive and innovate continuously.
Conclusion
In conclusion, while the challenges to AI integration are significant, they are surmountable with the right strategies and insights. By learning from industry leaders and understanding the transformative potential of AI, businesses can position themselves for success in this evolving landscape. The key lies in addressing common challenges, fostering a culture of learning, and embracing the future possibilities AI can provide.
What challenges have you faced with AI integration in your organization? Share your experiences in the comments below!